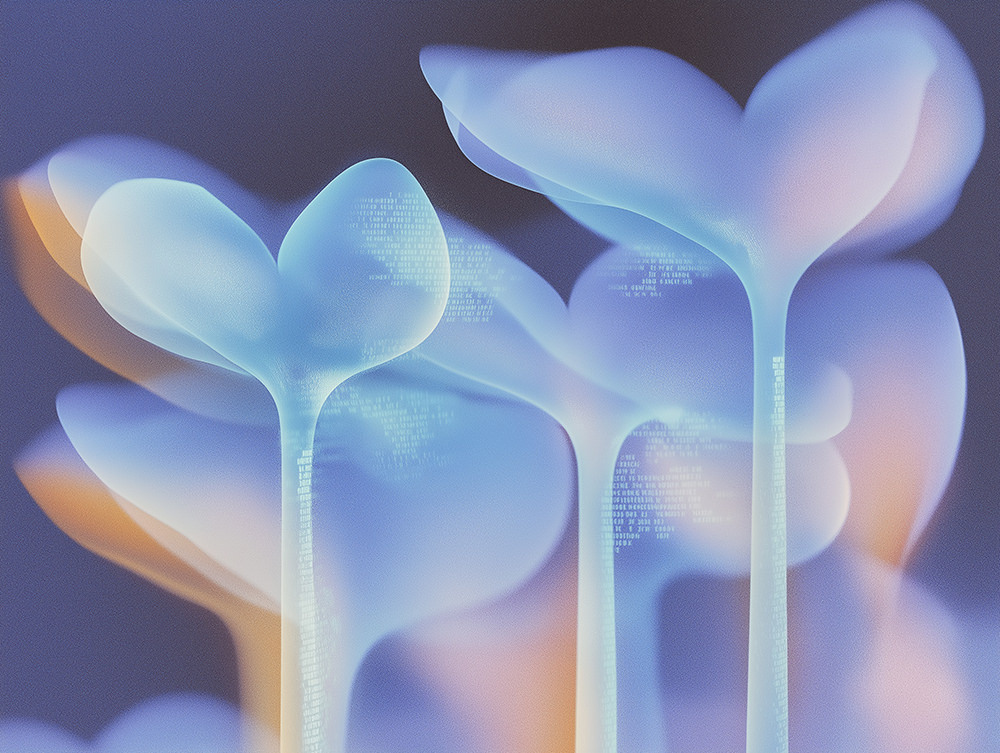
Selective State Space Models: Solving the Cost-Quality Tradeoff
As AI is increasingly used in production scenarios, costs are mounting. Are alternative architectures the solution?
It’s not always visible to end users, but machine learning (ML) has become a core component of every software product, influencing everyday experiences like food delivery pricing or ride-share wait-times. Training and deploying the models that power these use cases is challenging and complex, requiring tedious manual effort from data scientists. Tecton automates this complexity away from data scientists, helping companies operationalize machine learning quickly and reliably. The company is on track to become a core part of the emerging MLOps stack, helping engineers build ML into their applications.
We first got to know Mike and Kevin from their work at Uber, where they made significant contributions to Michelangelo, the company’s underlying ML platform. Uber, at the time, was one of a few companies able to operationalize machine learning at scale. Mike and Kevin realized there was tremendous demand for ML operationalization, and in 2019, started Tecton to enable more companies to harness the transformational power of machine learning. They created the feature management category, which has become an industry-standard approach to train and serve models.
To get a model into production, data scientists and engineers do massive amounts of data wrangling work to extract features from raw data. Then, they must store features so they can be re-used, keep features updated as new batch or stream data becomes available, and build feature pipelines to train and serve models. Tecton handles all of this for the customer, drastically accelerating a company’s journey towards operationalizing a model. Many companies ranging from small startups to large F500 corporations have successfully used Feast, the leading open-source feature store maintained by Tecton, and are now adopting enterprise-scale Tecton deployments to automate more of the ML workflow.
Today, Tecton is the cornerstone of ML platforms across a variety of companies and verticals, including Atlassian, Convoy, Rappi, HelloFresh, and more. Mike and Kevin have fostered an impressive community of MLOps experts, attracting over 10,000 attendees to their apply() conference. With Feast, the leading open-source feature store, Tecton has doubled down on its commitment to data scientists and engineers. Tecton is the leader in ML feature management and has been rapidly growing both its customer base and community.
We are thrilled to be working with Mike, Kevin, and the rest of the Tecton team by investing in their $100m Series C, joined by our friends at Kleiner Perkins, Snowflake, and Databricks. Machine learning is a must-have for every modern organization; Tecton is a must-have for every ML team.
As AI is increasingly used in production scenarios, costs are mounting. Are alternative architectures the solution?
Cube is the standard for providing semantic consistency to LLMs, and we are investing in a new $25M financing after leading the seed round in 2020.
In this edition of “In the Lab,” Amit Aggarwal explains why he’s building an AI startup in BCV Labs after selling his company The Yes to Pinterest.