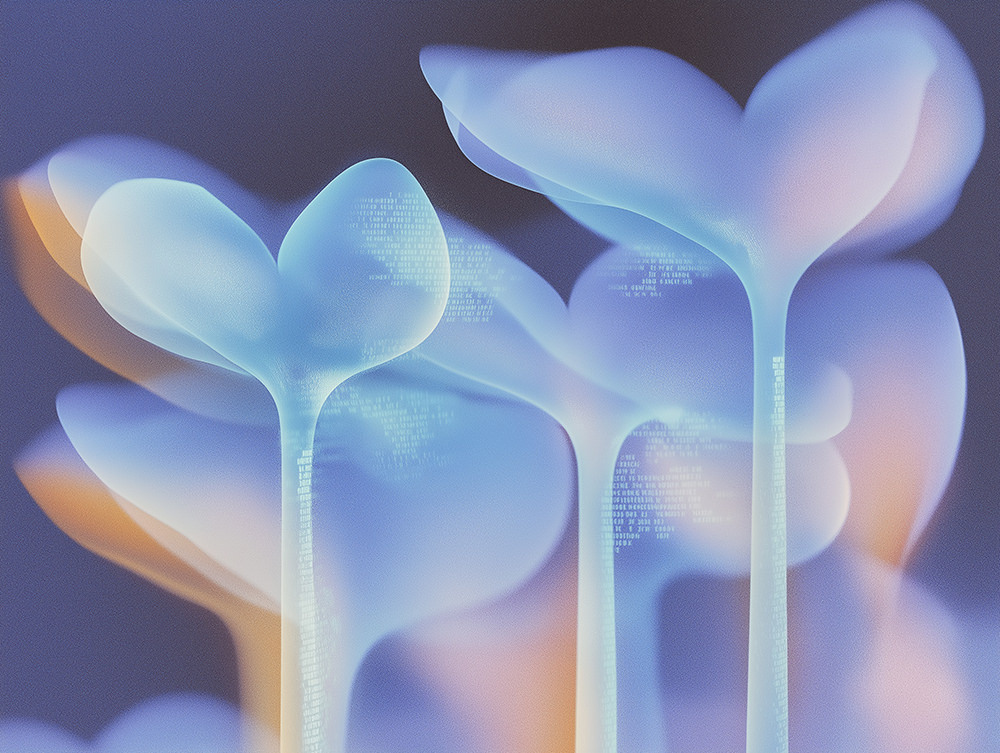
Selective State Space Models: Solving the Cost-Quality Tradeoff
As AI is increasingly used in production scenarios, costs are mounting. Are alternative architectures the solution?
Cube is the standard for providing semantic consistency to LLMs, and we are investing in a new $25M financing after leading the seed round in 2020.
Anyone who wears eyeglasses can relate to the feeling of putting them on in the morning: Previously fuzzy, amorphous, conflicting copies of information suddenly become clear; sharply structured and unified. Language models, and the applications built atop them, routinely suffer from blurry vision today. They have access to all of the information they need, but aren’t able to focus on the right source data to answer the right prompts.
Cube is building a pair of glasses for AI applications, making it possible for developers to define a data model once and consume it everywhere else in their application, while handling caching and governance from day one. As every application becomes an intelligent, AI-enabled experience, Cube has become a foundational layer of the underlying data stack, enabling more performant and secure data perception, transformation and reasoning, with minimal hallucination.
We led Cube’s seed round in 2020 and are excited to once again invest in the company as part of a $25 million financing. In the seed announcement, our partner Stefan Cohen wrote, “Cube abstracts away data-querying infrastructure into a simple API…[enabling developers] to query any data with low latency, flexible transformations and a consistent API schema.” Today, this approach has become even more critical, with users expecting AI copilots to retrieve and manipulate data in real-time, despite AI having high latency, a reliance on precise prompting, and vulnerability to inconsistent schema definitions.
When Cube started, the analytics stack was beginning to emerge. Since then, companies born in the cloud have scaled with large-scale OLAP systems like Snowflake, Databricks and BigQuery, while the rest of the world is migrating over.
Storing the right telemetry, logs, events, customer activity and more is hugely valuable, but introduces new complexity: an ever-expanding web of permissions, caches and metrics definitions (is “active_users” or “users_last_month” the right column for a MAU dashboard?). Cube abstracts away semantic complexity, helping people and LLMs alike navigate conflicting metrics, overlapping schemas and cascading permissions issues that plague every data fabric.
We’ve enjoyed working with the company over the past four years, as Cube made significant progress. Cube now powers data experiences at 20% of the Fortune 1000, and nearly five million developers are using it to build intelligent applications of the future.
Co-founders Artyom Keydunov and Pavel Tiunov have done a tremendous job of scaling a world-class team of technologists to realize their vision. Together, they’ve both created and evangelized the semantic layer category, winning companies of all sizes with their viral open-source project and rapidly growing enterprise product. We’re thrilled to support them on the journey ahead.
As AI is increasingly used in production scenarios, costs are mounting. Are alternative architectures the solution?
In this edition of “In the Lab,” Amit Aggarwal explains why he’s building an AI startup in BCV Labs after selling his company The Yes to Pinterest.
For now, most GenAI startups are focused on completing paperwork and are built on prompts. That may change in the months ahead.